What are Singaporeans' perception of surplus food and barriers to consumption?
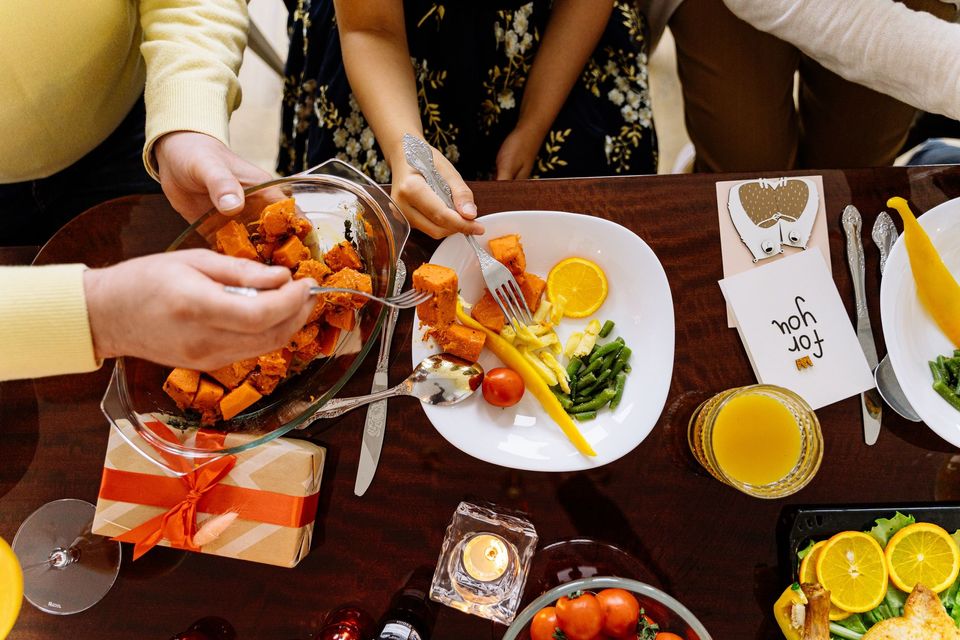
By the Kaggle Haggler team.
UPDATE 2021-12-25: This submission had unexpectedly won third place. Woo hoo! You can take a look at the post here.
📜 Problem statement
For this competition, we have chosen the third problem statement.
What are Singaporeans' perception of surplus food and barriers to consumption?
Our objective, therefore, is to use the dataset given to us by Synthesis to try to extract some insights on what people are talking about with respect to surplus food and food wastage in general. Another aspect to this question, if we go a little bit deeper, is on whether people are actually actively talking about the topics thereof.
📰 Background
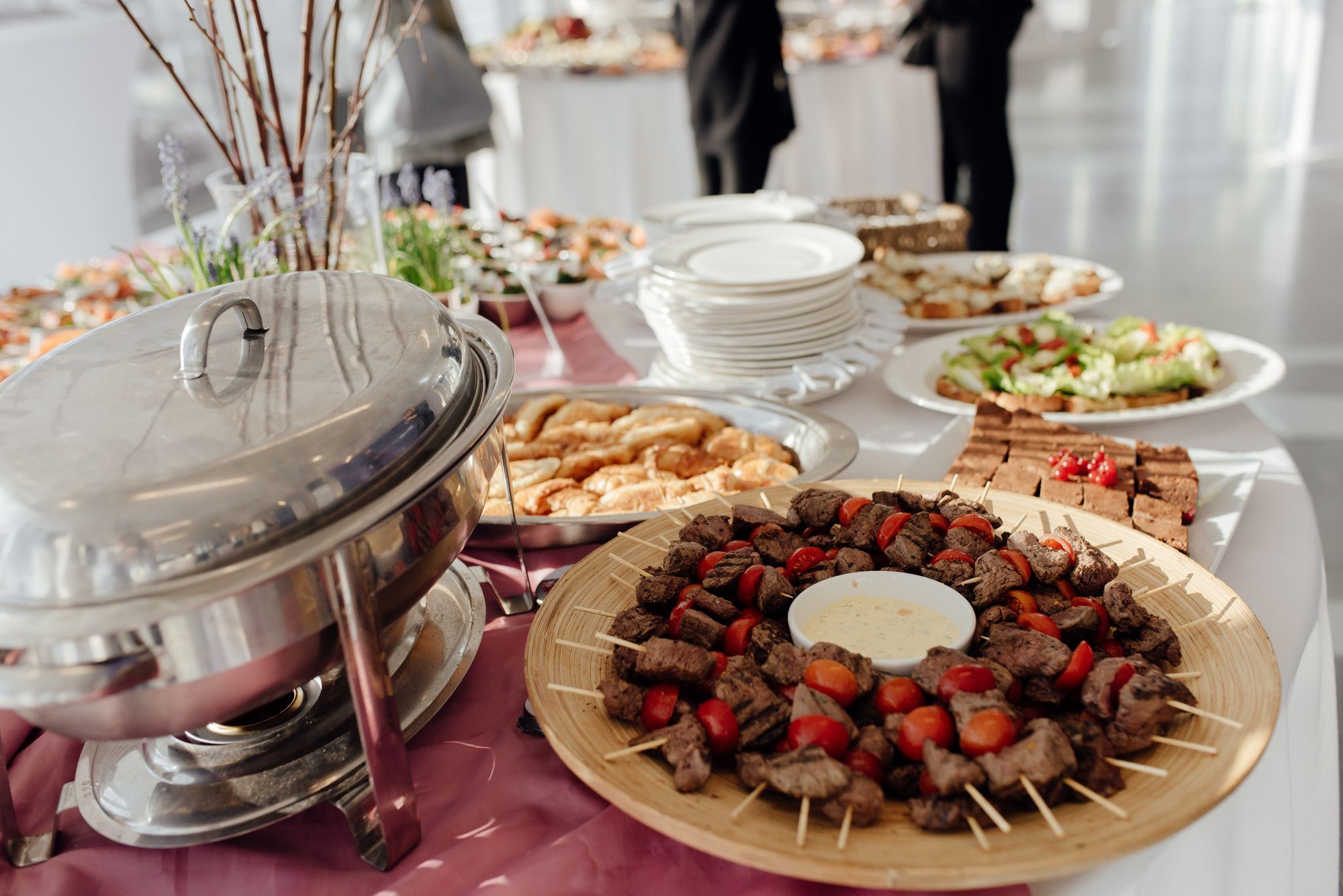
It is pretty reasonable to assume a certain consensus on the problems of food wastage and food surplus. The amount of food wasted grew by at least 40% between 2009 and 2019, though 2020—with the pandemic raging—showed a noticeable decrease in food wastage. That the amount of food is sufficient to feed everyone in Singapore should be a trivial fact to most people. Infrastructure, then, is the problem faced when referencing the issues mentioned. Limited efforts by the government in redistributing surplus food led to technology-based initiatives created by its citizens. One of them, notably, is the partner involved with Synthesis in this competition—Treatsure.
💿 Dataset provided
We were provided with datasets on:
- Top accounts sustainability audiences engage with.
- Sustainability audiences post data.
Both these datasets comprise of collected data from Instagram in the past five years and Twitter in the past one year. In this case, we will not be using all of them, but mainly data based on (2), since we will be looking into what people are posting moreso than the top accounts.
🐥 Exploring the dataset
👥 Demographics
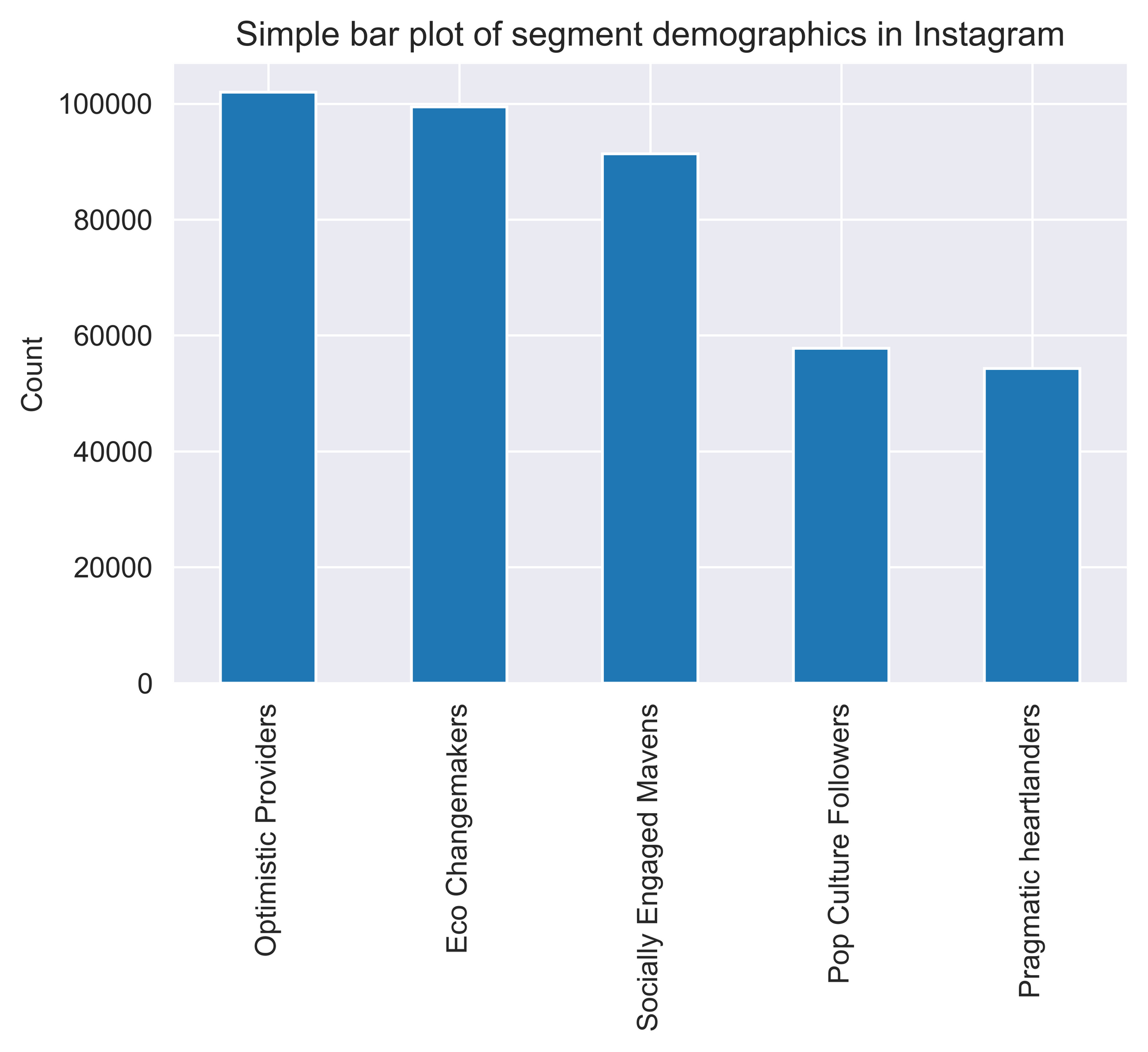
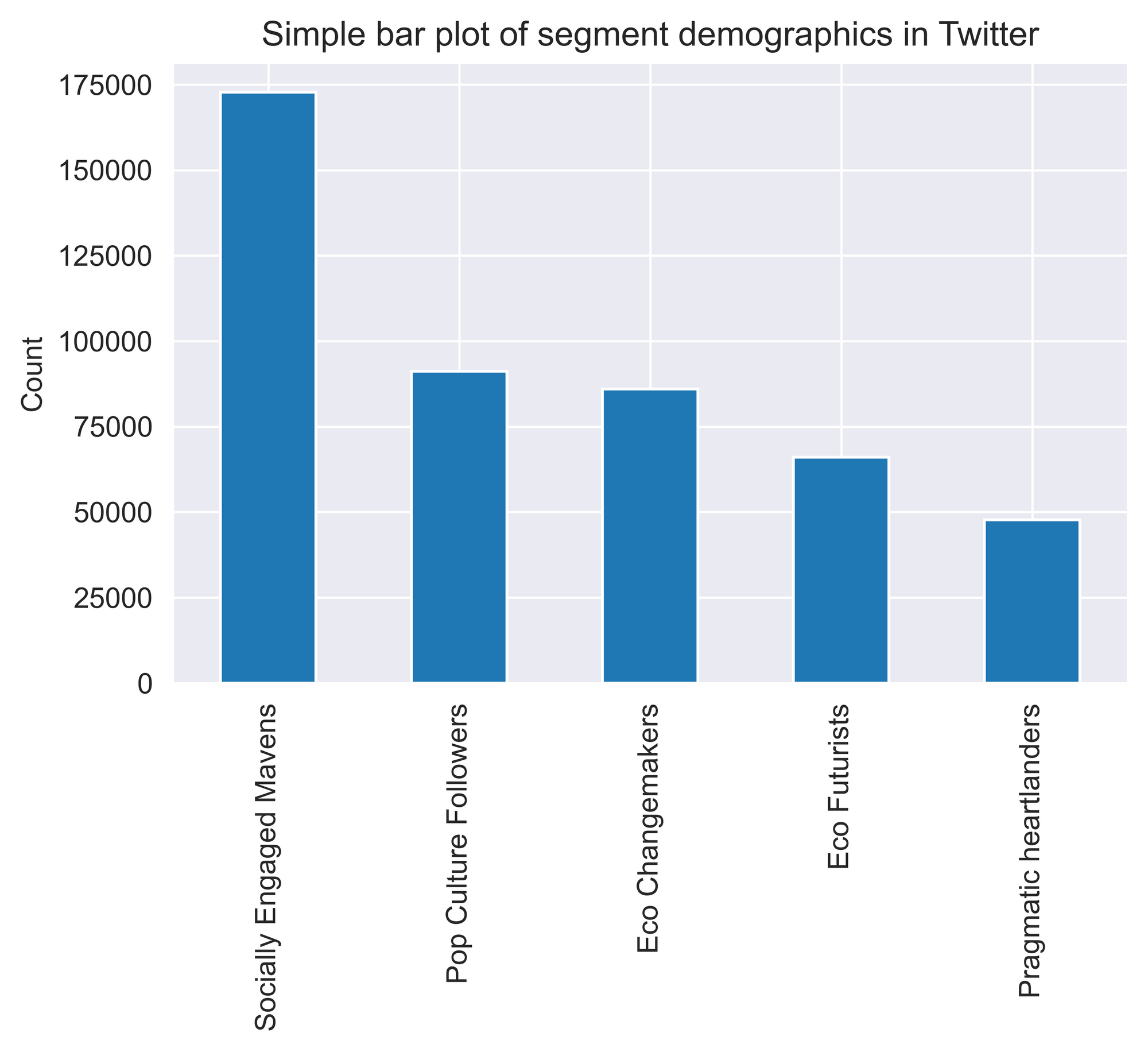
Interestingly, Socially Engaged Mavens and Optimistic Providers are the largest groups in the Twitter and Instagram dataset respectively, as compared to the population size provided by Synthesis with Pop Culture Followers as the largest. What would usually be the smallest group, Eco Changemakers, are at the top three in both datasets.
💬 How many times do people tend to post?
Some people tweet a little bit, and some tweet much more. We can see that there is a lot of variation in the amount of tweets and posts per user, and that is to be expected. But it does seem like 14000 Instagram posts per user over five years is a stretch.
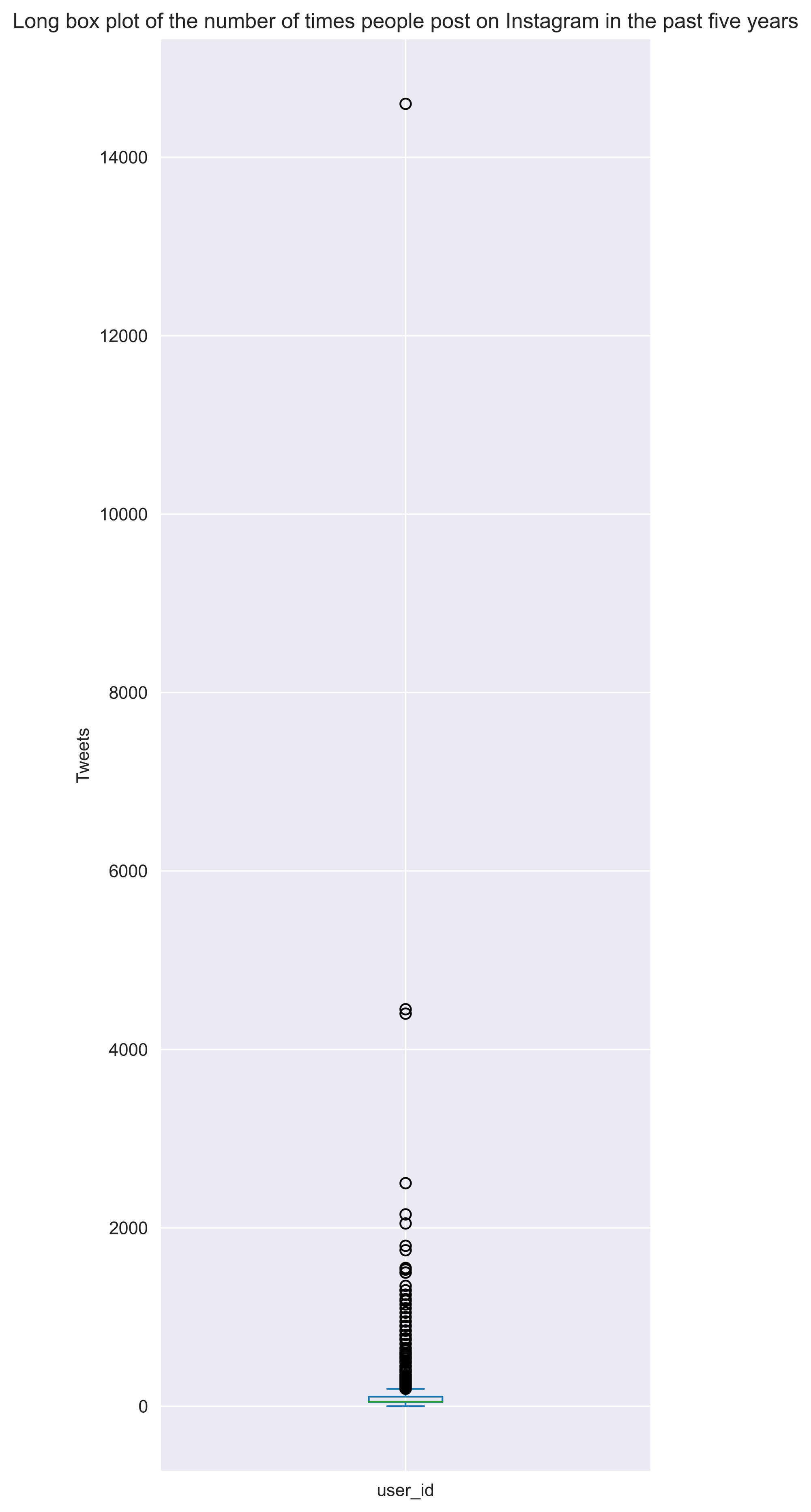
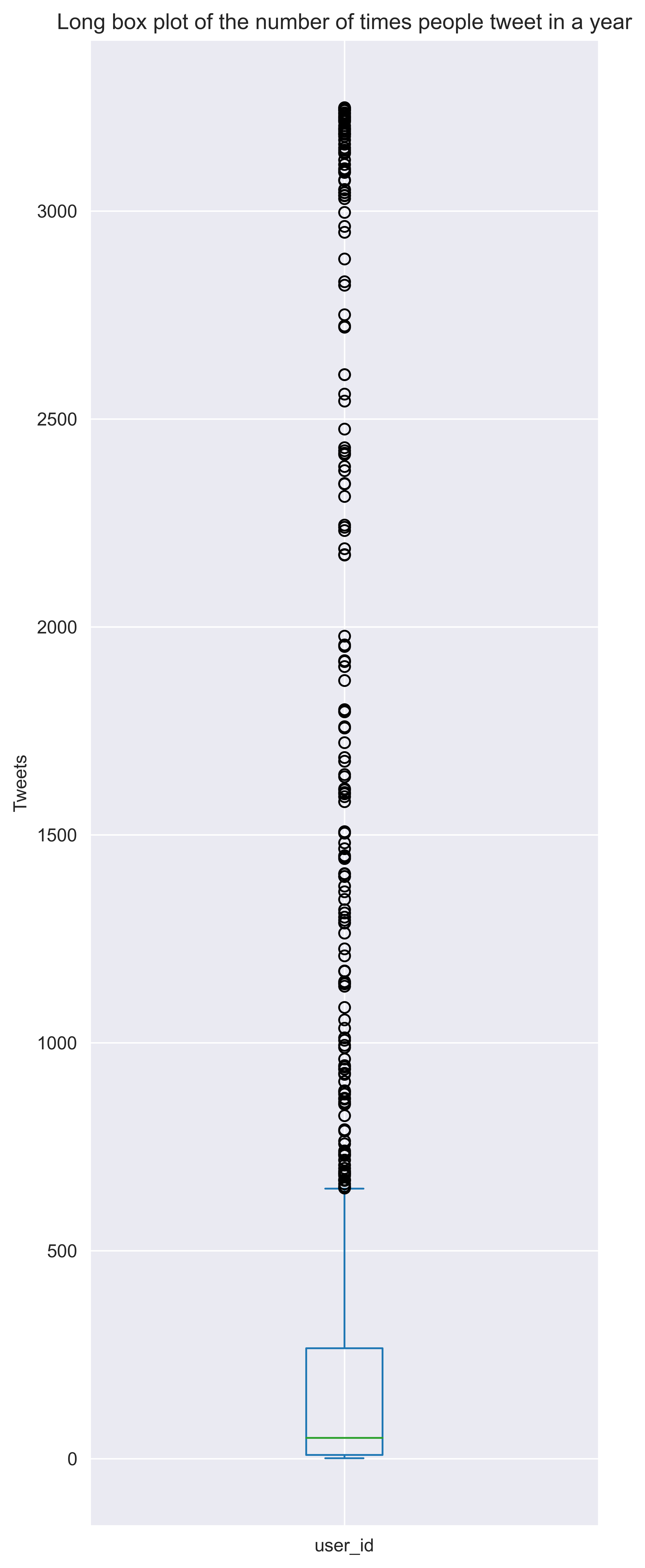
Naturally, we are curious to what people are typically tweeting about.
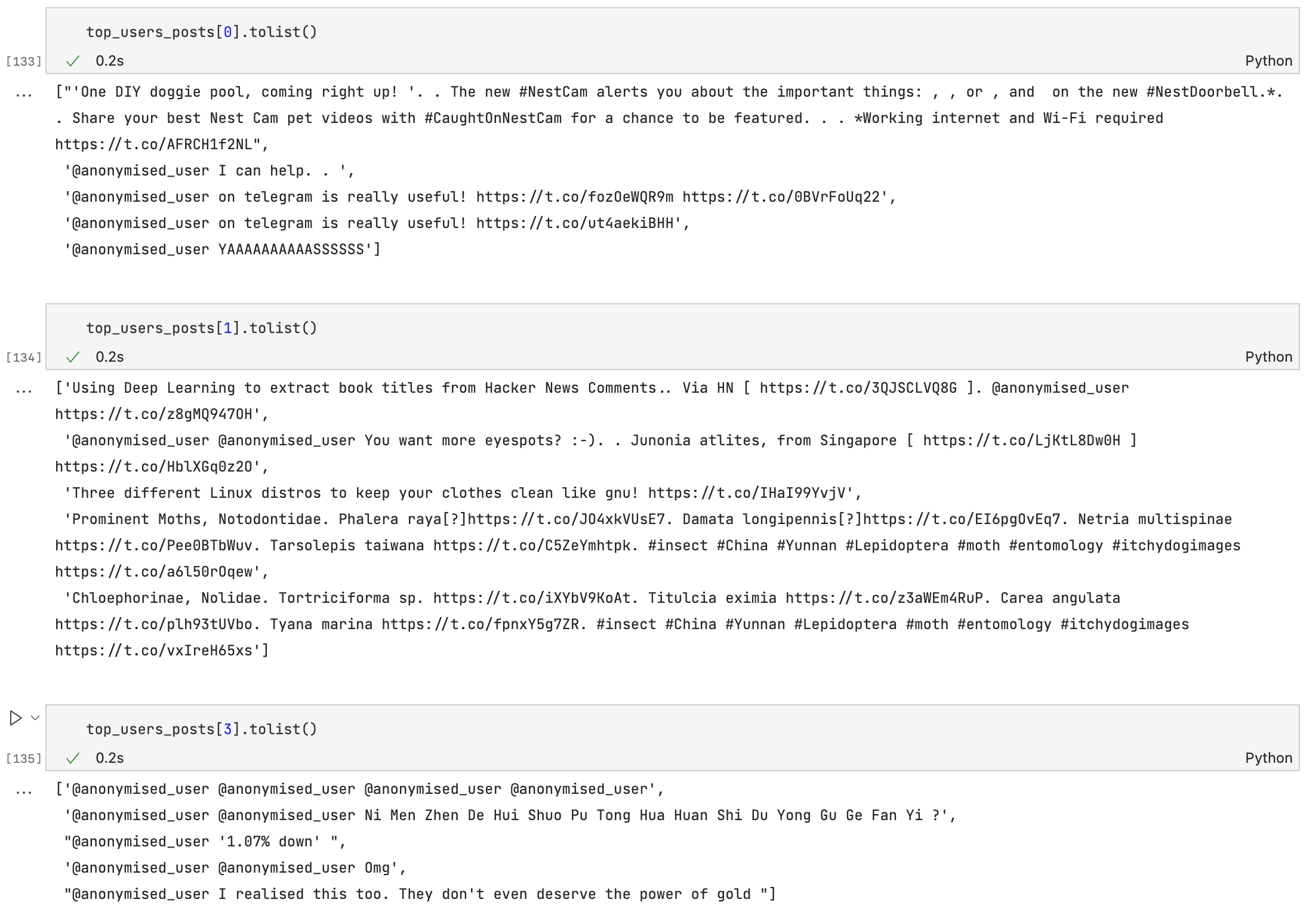
Seems like though they are tweeting a lot, most of them are not malicious or spammers or advertisers. Hence, we can keep the whole dataset.
For Instagram posts, it is a bit different. The top posters are mostly posting advertisements, and hence they are not that reliable for us. This makes sense as it is much less common nor socially acceptable to post many Instagram posts in a single day as implied by the amount of posts they have. Let us cut down the users who post more than 1500 posts over five years (this is arbitrarily based on an assumption that someone can post almost everyday for five years).
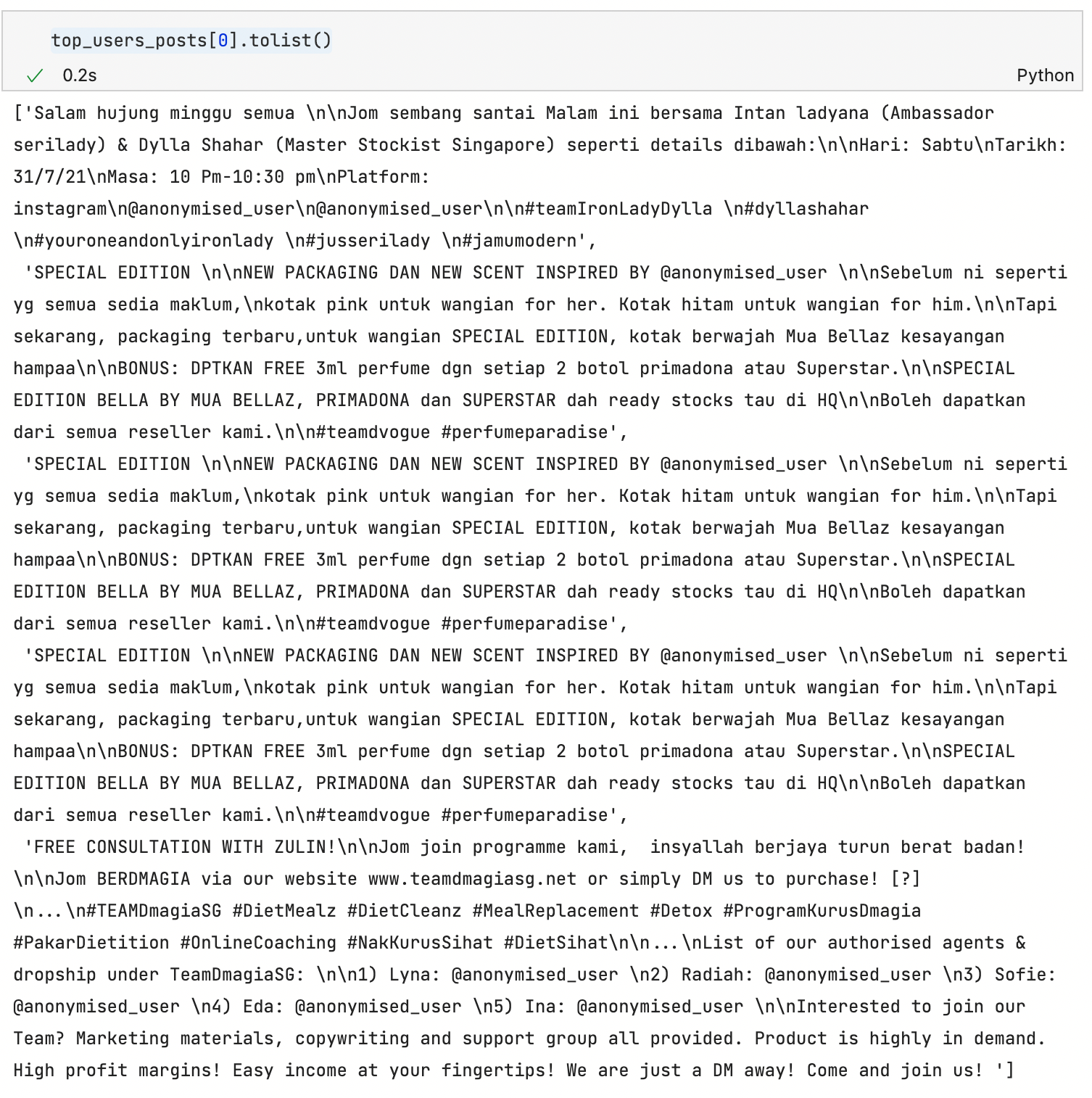
#️⃣ Hashtags
For tweets, the hashtags are not as prevalent anymore. We can see that out of all the tweets, only about 19% of them are hashtagged. Out of all of those, we can see that the most evoked hashtags are very current-affair-esque.
For Instagram, though, the numbers are significantly higher at 58.8% of posts having a hashtag. The nature of the popular hashtags are much more generic, however.
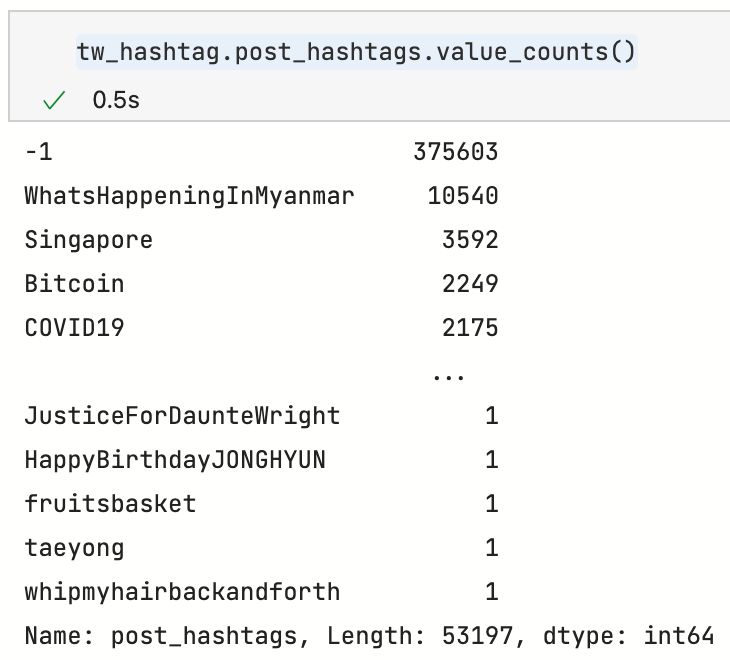
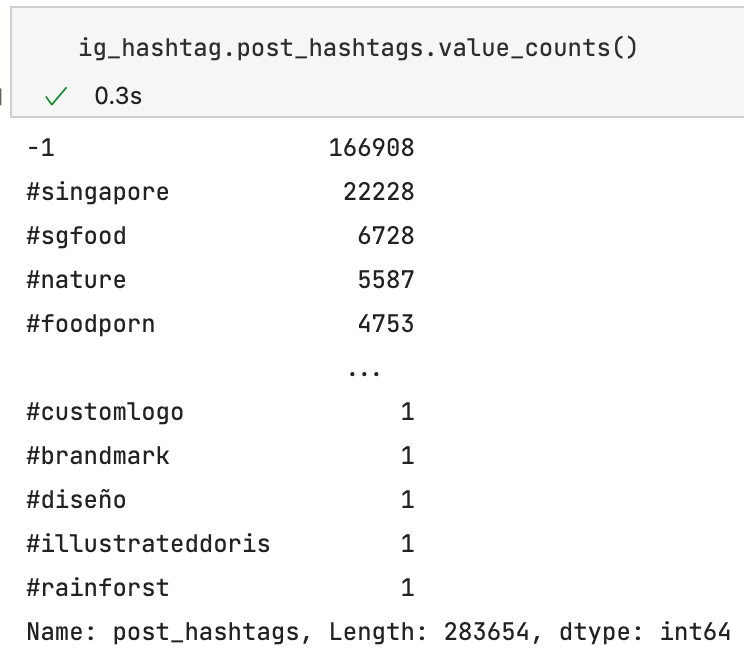
How about hashtags pertaining to food wastage or surplus, though?
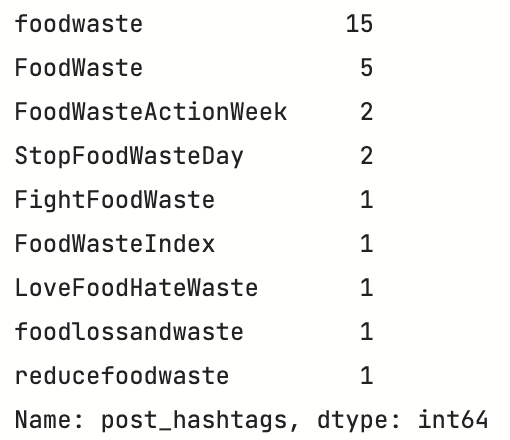
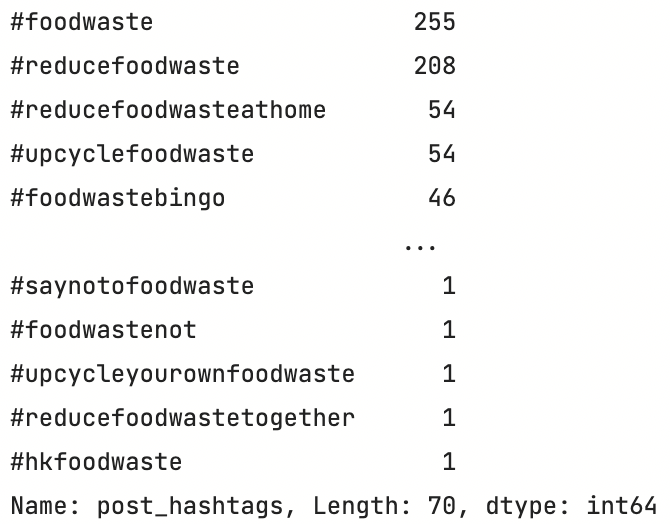
That is not a lot. But they might be useful, regardless! The demographics for the population filtered through these hashtags change, though in an expected manner. When we think about it, it is not surprising to see Eco Changemakers be on top in the filtered posts.
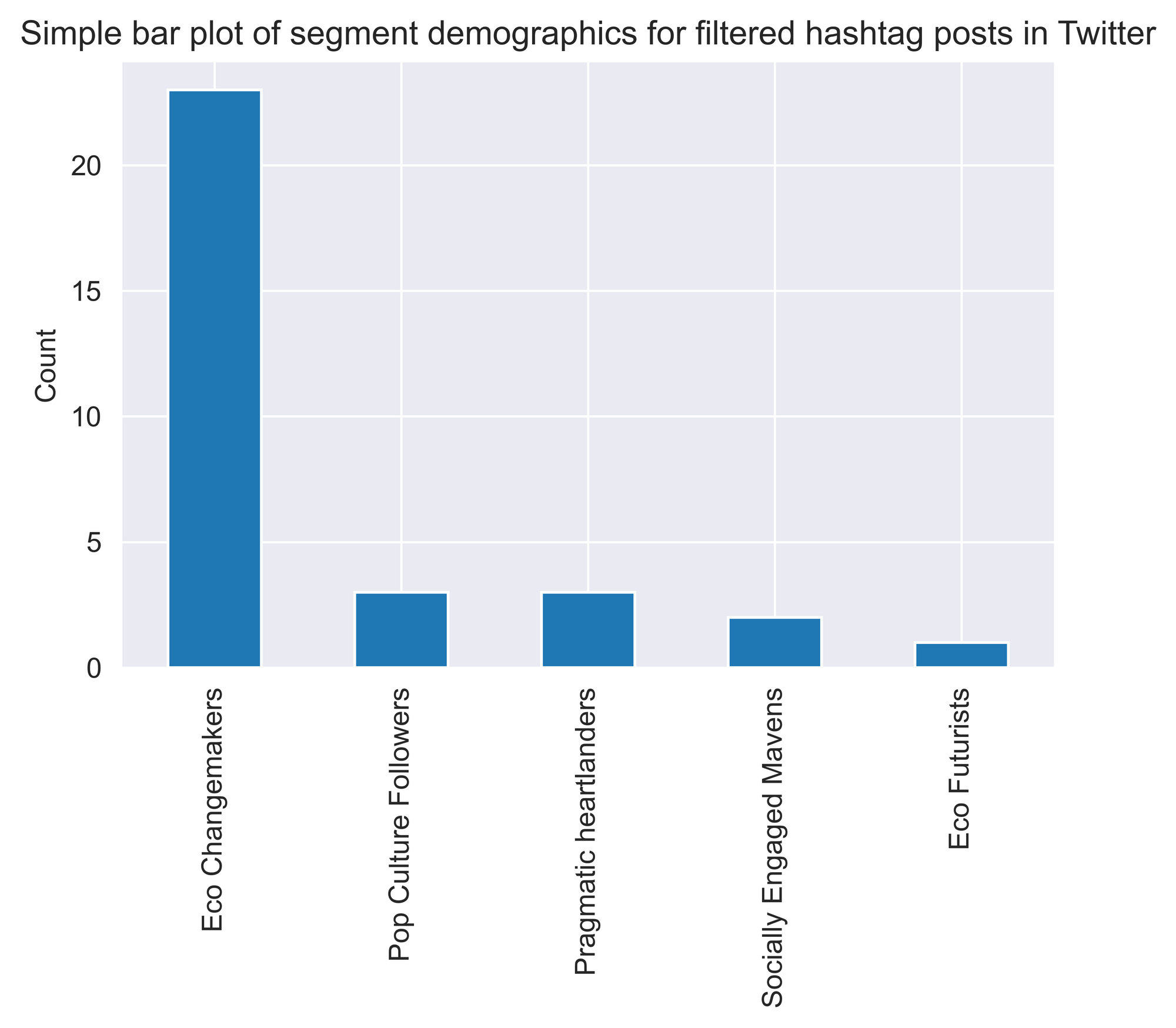
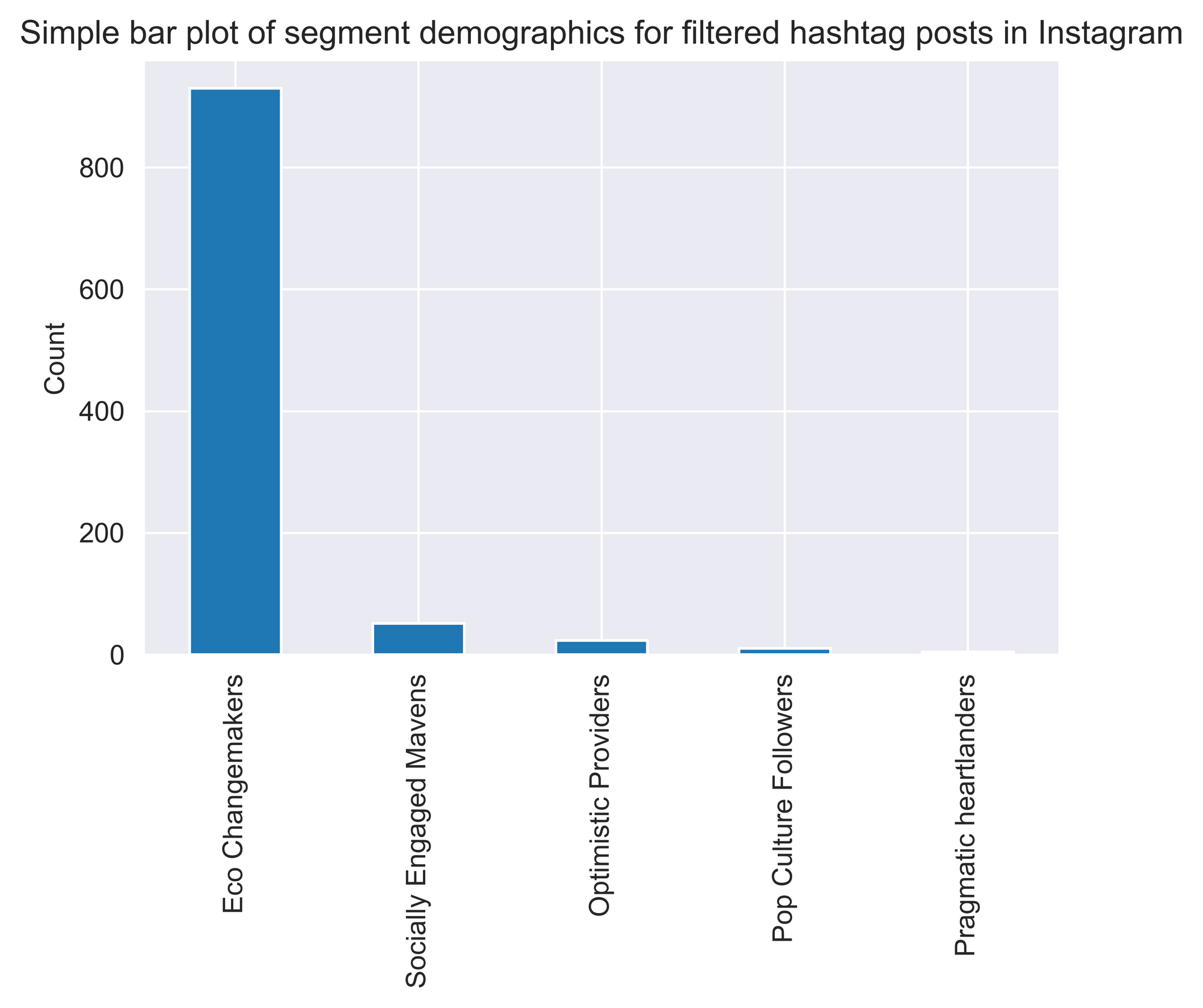
The Google Sheets frame below shows the kind of content these hashtags contain. Even if they are directly related to the problem statement, the goal of the tweets are more for raising awareness and engagements regarding this topic.
📂 Content filtering
We filtered the datasets for, first, food related posts and the from thereon, waste related posts. Based on this, we plotted the general trend based on year and segment. The keywords we used for food related posts are shown below.
searchfor = [" food "," eat "," makan "," steam "," boil "," ingredient "," roast "," chicken "," beef "," pork "," rice "," noodle "]
On Instagram, there was a general trend that most people posting about food waste were Eco Changemakers and there is a general increasing trend of posts about food waste year on year, especially from 2019 onwards.
Interestingly, on Twitter, there were more Socially Engaged Mavens. This could be because of the use of the retweet function on Twitter. However, as there is only one year's worth of data, we cannot really comment on the year on year trend in number of posts.
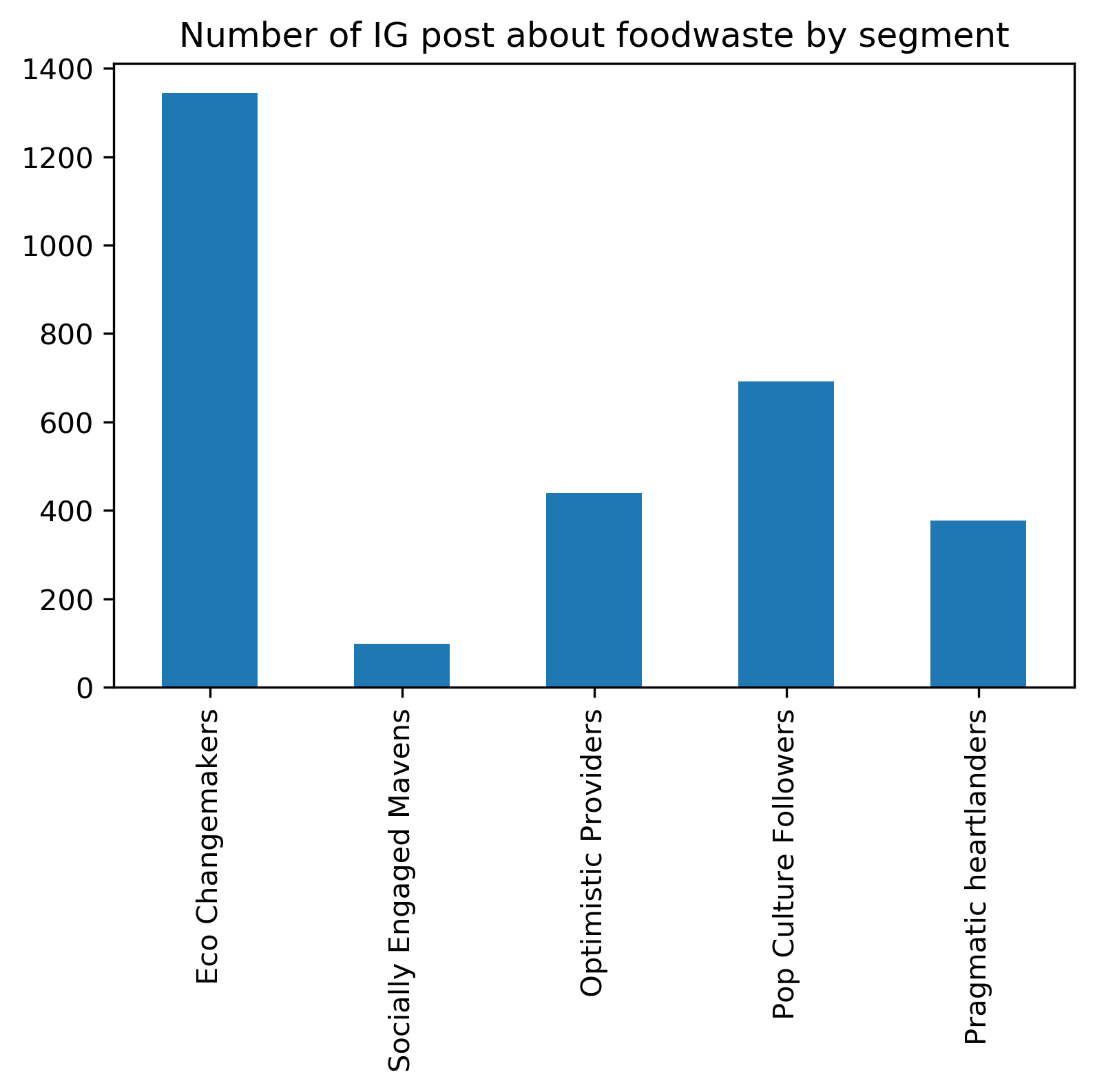
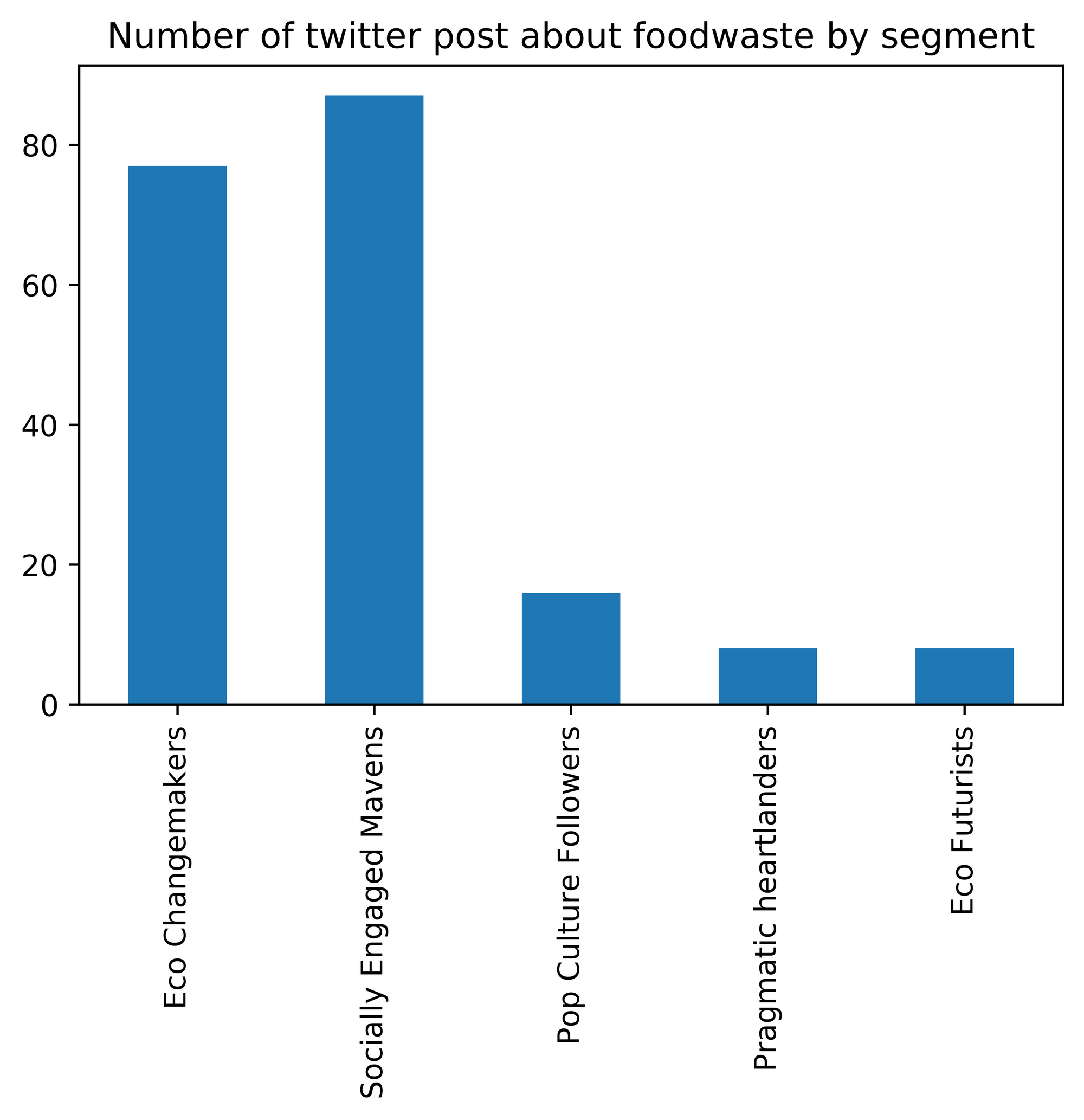
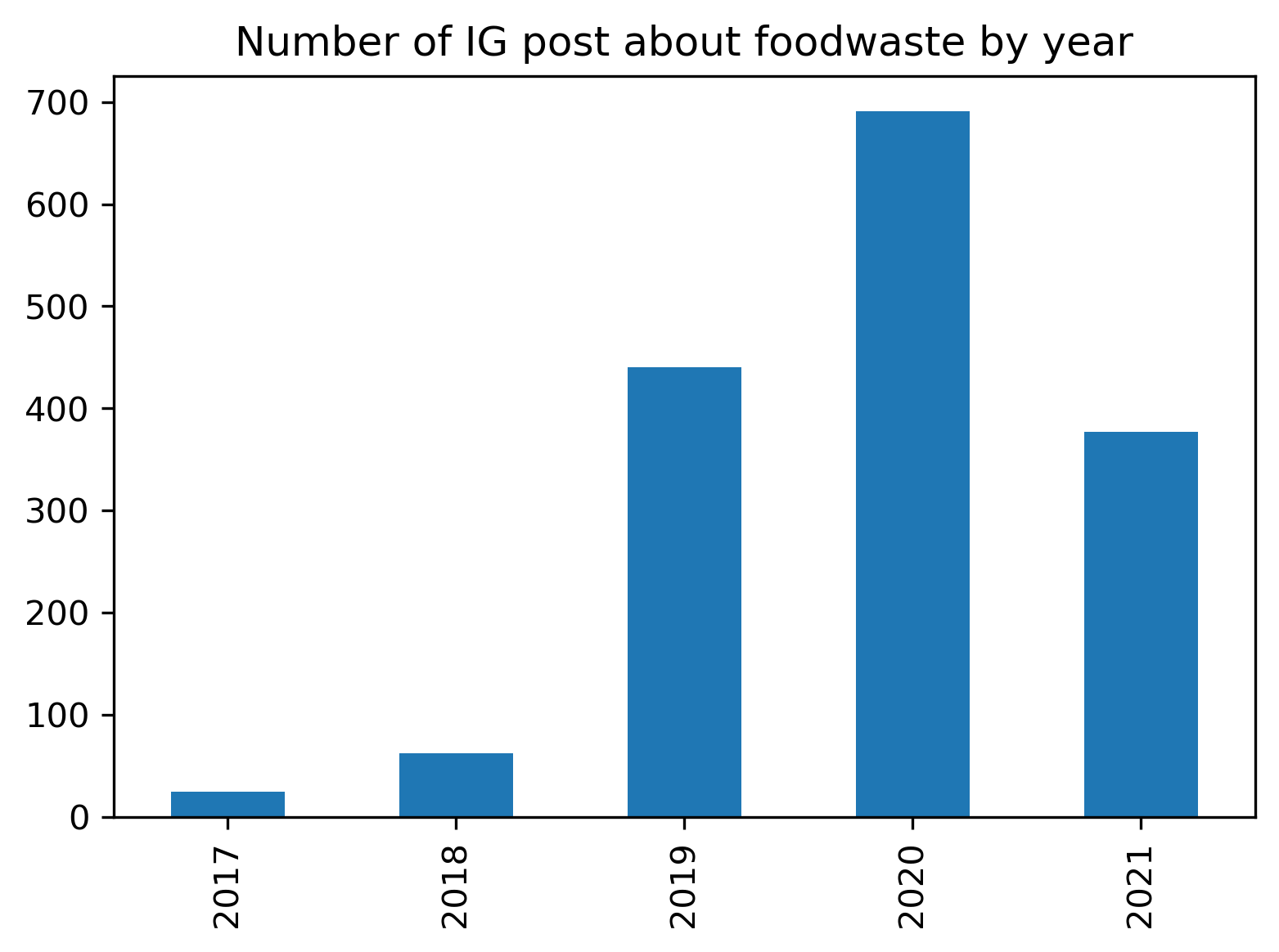
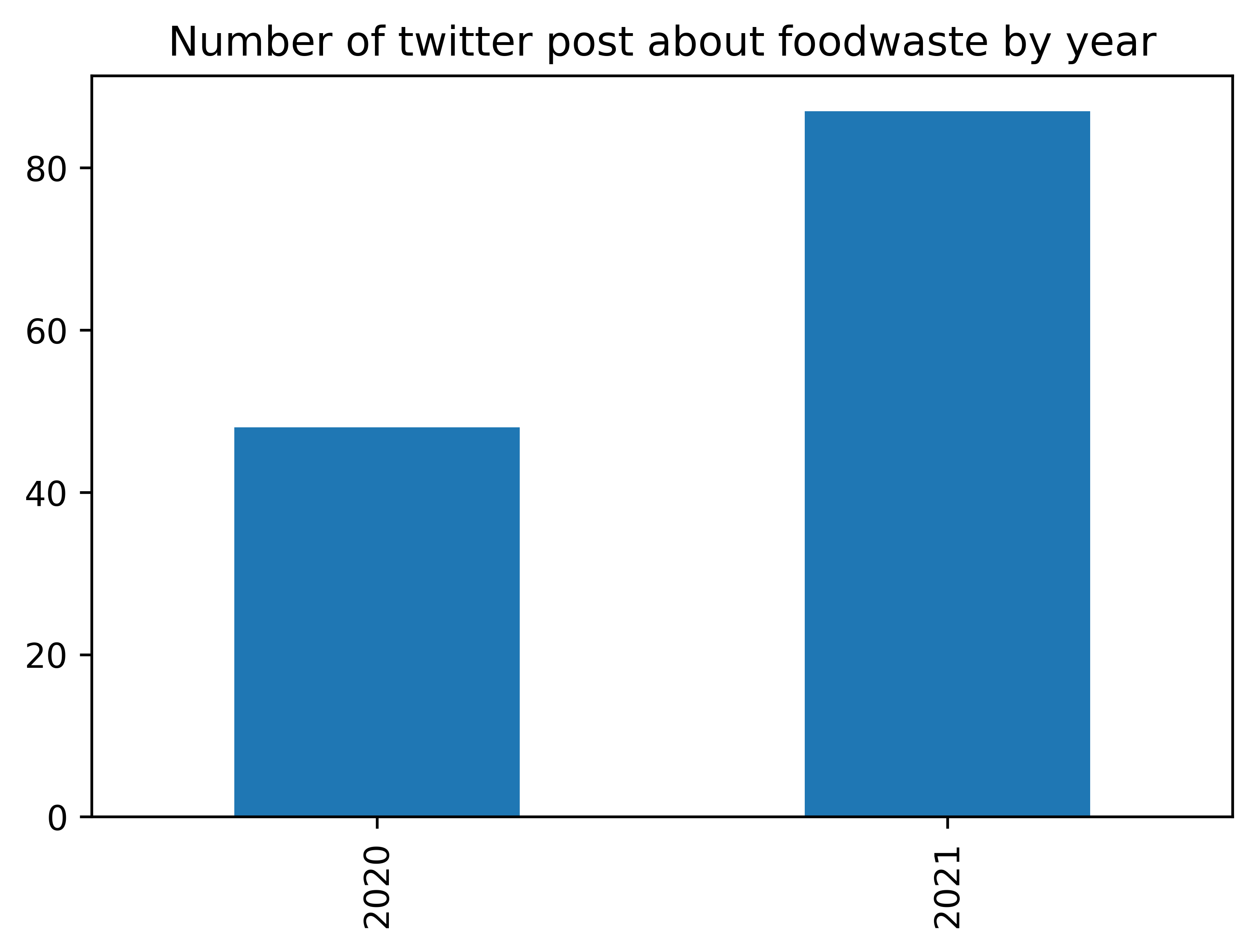
🕺 Models
😶 Finding word associations
In this case, we used Word2Vec to learn word associations within each corpus from the two datasets. It is a shallow two-layered neural network to perform word embeddings. The word vectors are then projected into a t-SNE graph which approximates clusters of words near each other. The closer they are, the more associated the words are semantically.
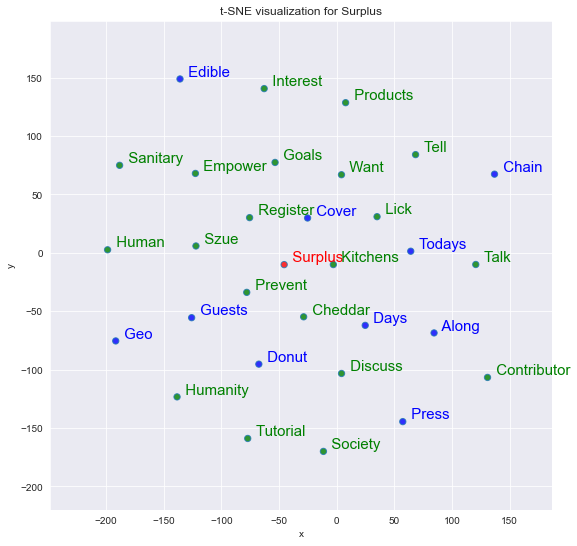
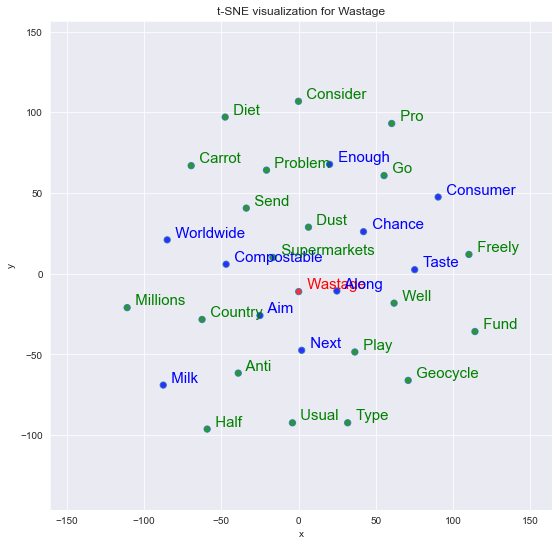
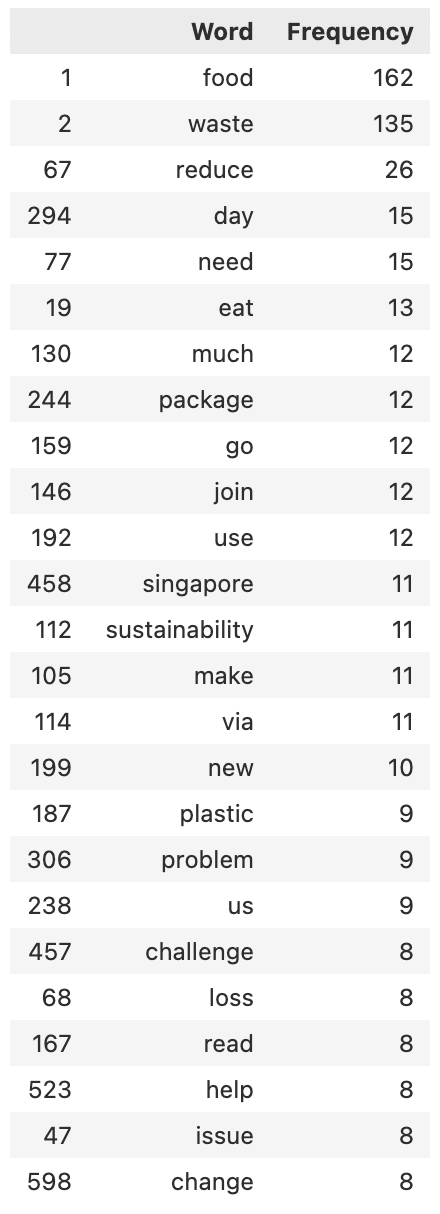
In this case, looking at words most similar to Surplus and Wastage gives a bit of an insight to some keywords considered by people when talking about them. This includes the words 'sanitary', 'edible', 'compostable', 'empower', and 'taste'. These can be a good place to start when judging people's perceptions on surplus food and the consumptions thereof.
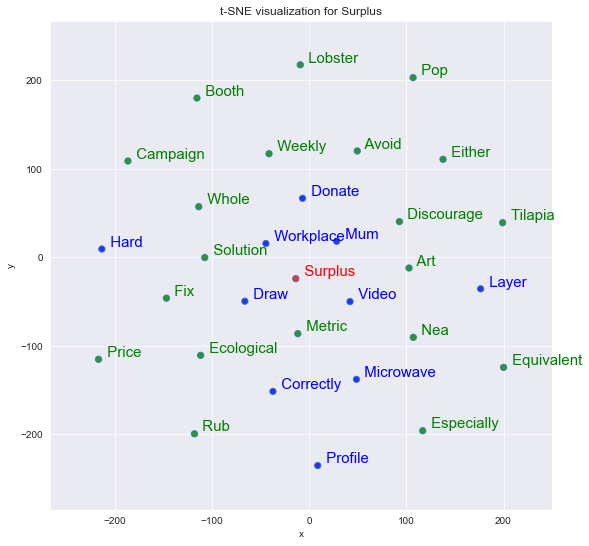
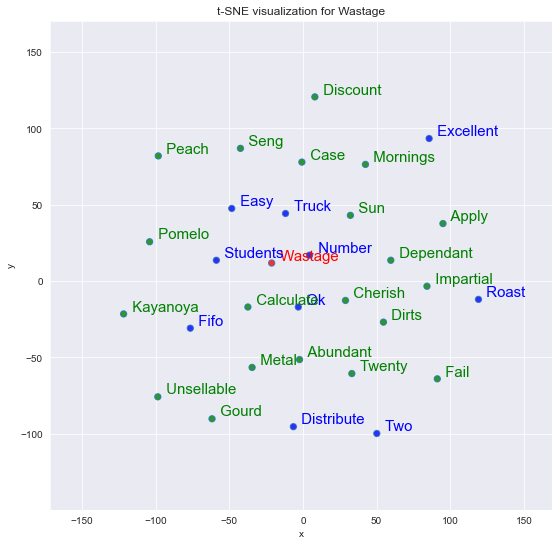
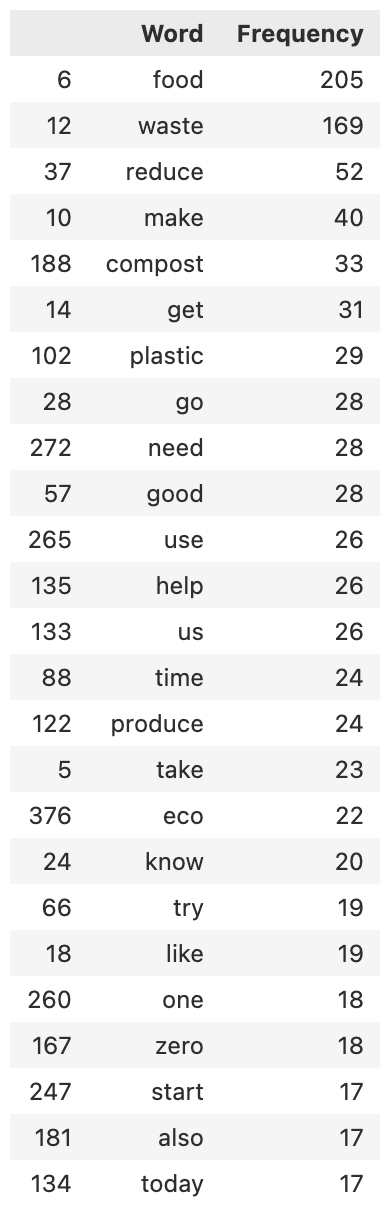
Here, we see a different set of words, including 'donate', 'avoid', 'microwave', 'ecological', 'unsellable', 'discount', and 'distribute'. It is a bit more of a mixed bag in terms of the sentiments of the words seen here, but we believe that it has the potential to be as useful too.
However, we would like to also note that the method might not be the best in finding exactly what people are talking about with respect to food waste and surplus, since this model is very word-based. Also, the relatively small corpus sizes was also a limitation for this model, as can be seen by some irrelevant words in the graph.
❤️🩹 Sentiment analysis
The content filtering gave us some insight as to how Singaporeans' awareness of food surplus and waste developed over the years. However, although there seemed to be an increasing trend, we were curious about the nature of this growing awareness. Were people more concerned? Or did they think the topic was over-hyped? How were their habits of curbing or enabling food wastage changing with the opinions?
With these guiding questions in mind, we decided to utilize sentiment analysis to help us better determine the sentiment behind it. The sentiment analysis model we used outputted two values, polarity – referring to the intensity of emotions expressed in the sentence, and subjectivity – referring to one's personal feelings, views, and beliefs.
Next, we compared the polarity over the different segments to see if certain segments tended towards more positive, neutral or negative sentiments about food. From the bar chart on the left, we can gather insight as too how prevalent the topic of food waste is to each segment. For example, given that Socially Enabled Mavens and Eco Changemakers are the two smallest demographics, the fact that they have the highest number of Instagram posts indicate that this is a topic often discussed and shared about. On the contrary, we can posit that food waste is perhaps not something often on the minds of our pragmatic heartlanders, given the few posts.
Normalizing the sentiments allow us to more clearly compare within each segment, the proportion of positive and negative sentiments. Generally, they seem to align with our expectations, with Eco Futurists having most positive sentiments, followed by Socially Enabled Mavens and Pragmatic Heartlanders. The surprising thing was how poorly Eco Changemakers seemed to perform, and we attempt to rationalize this in our limitations below.
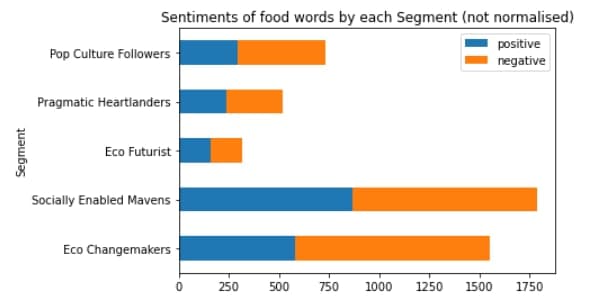
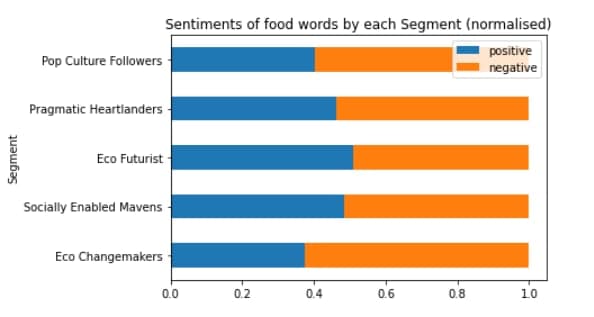
😥 Limitations
A limitation we found doing sentiment analysis based on the posts, is that they can hold similar view but have different sentiments. Below is an example of a negative sentiment and a positive sentiment, both expressing views that encourages not wasting food.
Negative sentiment: “I hate when people waste food”
Positive sentiment: “I enjoyed eating my leftovers”
One possible way of discerning could be training our own model, however in view of time, we were unable to do this.
Tying back to our attempts at understanding the sentiments of our segments, it is possible that Eco Changemakers tend towards more negative language, for instance expressing stronger opinions about other's habits rather than affirming words about their own.
Another limitation was that we found most post were in support of reducing food waste or eating surplus food. This is likely because it is the more politically correct view, and people are more likely to be posting asking others to eat their surplus food than to share about how much food they wasted. This is especially so given the sites chosen, Instagram and Twitter. Instagram is place for validation and although twitter has more anonymity, is also a place people go to seek validation and find community.
People do tend to post their controversial views online, but we believe these posts are rarer (and the social media sites chosen might not where controversial posts are often posted) to come about. Perhaps these social media blogging sites are not the best place to find barriers to consumption.
If given more time we would advise looking into article posts, Facebook comments or using sites with more anonymity like Reddit.
📝 Syntax analysis
In order to extract people's reasoning for their behavior, we decided to study the syntax of the sentences and extract sentences where there is likely to be a rationale for their actions or decisions.
In order to do this, we came up with out own sentence with hypothetical barriers to consumption. We used this to look at the syntax of sentences explaining rationale.
The sentences used was: "I hate extra food because they taste cold. Hmmm well I guess I could try."
Although not perfect, we found that a preposition followed by a verb followed by a adjective can sometimes show a rationale.
Example of a reason: “they(prp) are(v) cold(adj)”, however, it is limited as this same syntax also appears when someone is talking about an action and are more descriptive with their nouns. “I(prp) ate(v) delicious(adj) vegetable(n)”
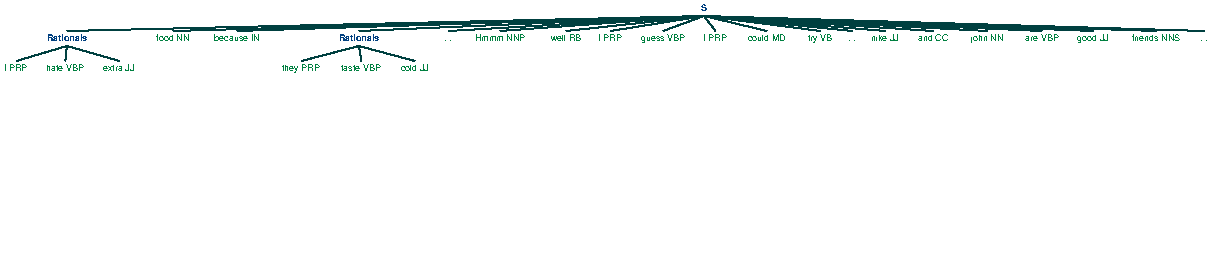
This method can be refined by perhaps using information from linguistic to better understand language patterns. However, in the view of time, we have went ahead with identifying this pattern, because even though the second example is not exactly an explanation, the adjective can also be informative. From the sentence I ate delicious vegetable, it can be implied that the reason they ate the vegetable is because it is delicious.
We filtered the posts and any sentence with a phrase that contained the syntax mentioned above was extracted to be studied. One of them is shown below.
One of the biggest #zerowastetravel challenges is FOOD WASTE. 😞Everything looks so bloody delicious and you might never go back, so why the hell not right? 😳 #foodiepains #cannotzipmypants 😫 These are some hacks I have for minimising #foodwaste on the road.☝🏻Pack what you can't finish (portioned nicely of course!) and give to someone who might appreciate a warm meal, or save it for late night munchies. (Bonus for #BYO bags!! 🙌🏻)✌🏻Ask if shops might have tasting samples (instead of buying full portions that you can't finish) - you might get lucky! 🤟🏻Ask fellow diners if they'd like to try any of the food on your table (BEFORE you go HAM on your food!) - opportunity to make new friends! Travellers have the same problem of not being able to stomach all the food they wanna try, so there's a good chance your offer will be welcomed. #pickupline #sharingiscaring 😉 #mindfulconsumption #feedpeoplenotbins #zerowastetravel #zerowastejourney
We then went through these posts to find patterns and insights regarding surplus food consumption. In the case above, the idea we got is that once they start eating the food, it is deemed as messy, and thus they feel embarrassed to ask other people if they want it.
💡 Insights
Based on the rationale sentences found in the syntax analysis, sentiment analysis and word associations, we found some factors encouraging consumption and acting as barriers to consumption.
☑️ Encouragement for consumption:
🌎 Environmental concerns:
- Carbon miles of food are very high.
- Food waste in landfill rots and produces methane a greenhouse gas.
- People are generally very concerned on social media about sustainability.
👥 Social concerns:
- If food is not wasted, people would not have to go hungry.
- A general desire to donate surplus food to people with food insecurity.
💸 Cost savings:
- Hopefully to save some money.
- Compostable consumables can be cheaper and very beneficial to plants.
⛔️ Barriers to consumption:
- Do not know the difference between food scrap and food waste.
- Do not want to consume food that are not tasty or perceived to be not tasty.
- Unsure if the food is spoiled or not.
- Half-eaten food is unsavory, embarrassed to share, not willing to take as it is deemed as unsanitary.
- Expecting surplus food to be cheap or cheaper.
- It might be that food-sellers consider their surplus to be unsellable too.
😶 Sentiments
Eco Futurists have the most positive sentiments, followed by Socially Enabled Mavens and Pragmatic Heartlanders. Eco Changemakers seemed to perform poorly, but we covered the limitations of our model in the previous sections.
✍️ Dataset quirks
We also found, as seen in sections before, that the datasets themselves do not contain as much opinions regarding food wastage. Even if they are talking about it, it tends not to be about its consumption. This might be an indication that a general awareness is lacking (as seen from the multitude of posts on raising awareness), and that the issue does not persist as much in people's minds. Hence, though we can find some form of insights through so-called 'eye-balling' the data a little bit, other sources of data might be better to find valuable opinions on why people are consuming or not consuming food surplus items.
One place we considered was Facebook comments on posts by news channels regarding food surplus. However, trying to scrape Facebook at our current level led to us either being blocked, or that it will take way too long to scrape. Hence, we did not do that, but rather consider that as a future improvement in order to be able to build great models for it.
Another area is on data labelling. This is a tricky problem as it takes a lot of man hours to do so. Currently, the labels we have are for their segment names. However, it would be much simpler to create classification models if we have some labelled data to train on.
👋 Final words
We are all beginners to data science, and though this analysis is far away from perfect, we felt that we have learnt so much through this competition. We are especially very new to processing words instead of numbers, and it really pulled us down into deep rabbit-holes, be it in linguistics or word embeddings or sentiment analyses.
For the source code of our project, we are in the midst of compiling them and we will update the page with the GitHub repository for it.
Thank you for reading our submission!